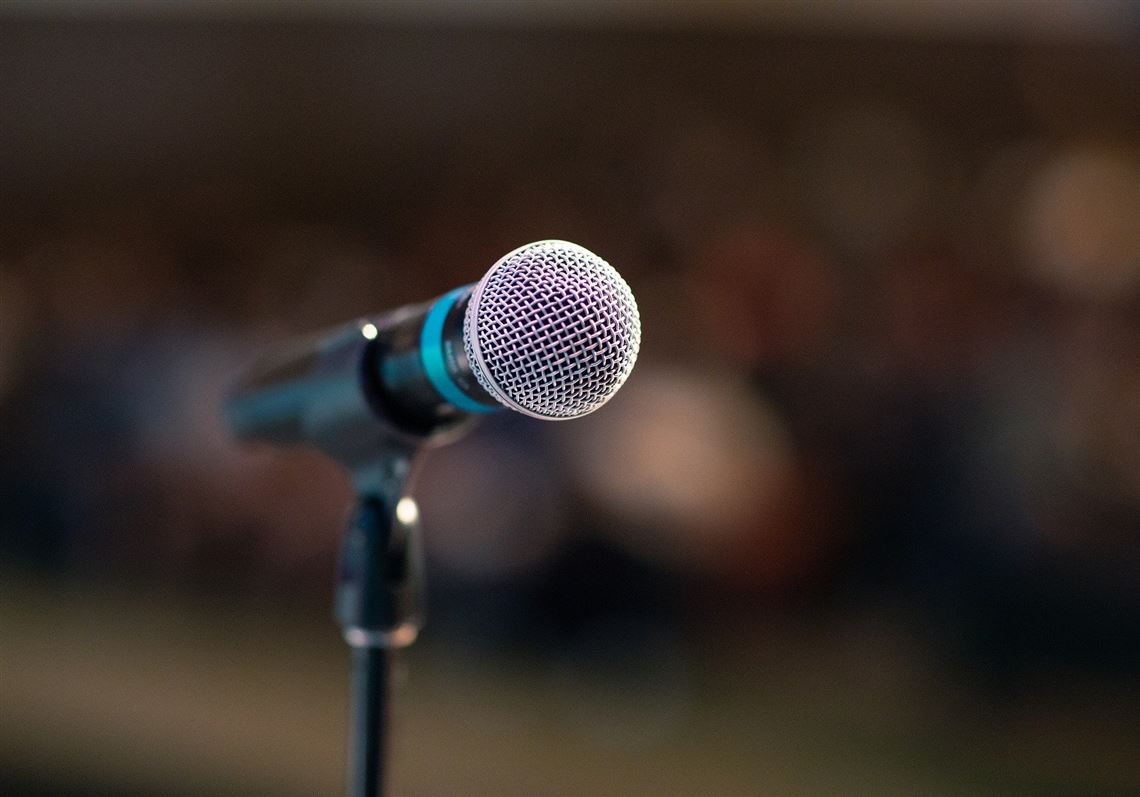
In our current peri-COVID world, we all now have far more experience than we could ever have imagined in remote working.
Our homes are now our offices; dress codes have become more relaxed; we can work somewhat more flexible hours to accommodate our personal lives.
This has all come at a cost, of course. The biggest, in my opinion, is the need for higher bandwidth and more reliable Internet connections to our homes. In many cases, Internet Service Providers (ISPs) have been hard-pressed to provide new pipes, and “last mile” service installations have lagged.
The Internet core network has similarly been stressed–in analysis done comparing pre- and peri-COVID data in several cities around the world, backbone data usage has gone up by as much as 40% year-to-year.
Much of this “need for speed” has been driven by widespread use of teleconferencing software. Zoom, Microsoft Teams, Skype, Chime and others are in constant use around the world. Even with clever bandwidth-saving measures, the massively increased use of teleconferencing has created what will probably remain with us post-COVID.
One of the contributors to the need for higher bandwidth in teleconferencing is the requirement to transmit timely and clear representations of speech in a digital format. Generally, audio is highly resistant to most compression technologies–it’s too full of unpredictable data patterns and, with noise added in, becomes even more of a problem.
A number of coder/decoder algorithms have been invented for the problem of transforming speech, in particular, to a digital form. Some are very clever, making use of models of speech generation to build compression models that are reasonably efficient of time and bandwidth. The models are made much more complex by the need to model a wide range of languages–many of which have substantial differences in their phonemes. Add in accents, speaking rate, and other variables and the models become extremely complex.
With the long history of language coder/decoder research, it would be easy to believe that there would be nothing new under the sun.
And that would be wrong.
Google has announced a new speech coding algorithm that appears to use much less bandwidth than existing algorithms, while preserving speech clarity and “normalness” better.
The new algorithm, named “Lyra”, is based on research done on new models for speech coding, generative models.
These shortcomings have led to the development of a new generation of high-quality audio generative models that have revolutionized the field by being able to not only differentiate between signals, but also generate completely new ones.
One of the major issues with using these generative models is their computational complexity. Google has offered a solution to that problem and the solution appears to offer better performance, at lower bandwidth, and with better apparent normalness to the sound quality.
Lyra is currently designed to operate at 3kbps and listening tests show that Lyra outperforms any other codec at that bitrate and is compared favorably to Opus at 8kbps, thus achieving more than a 60% reduction in bandwidth. Lyra can be used wherever the bandwidth conditions are insufficient for higher-bitrates and existing low-bitrate codecs do not provide adequate quality.
The Google webpage announcing this news has examples of their algorithm in action compared to existing, widely used algorithms. The results are quite impressive.
What impacts will this have on teleconferencing? Google predicts that it will make teleconference possible over lower bandwidth connections, and provide an algorithm that can be incorporated into existing and new applications.
Google plans to continue work in this area, most importantly to provide implementations that can be accelerated through GPUs and TPUs.
Be sure to listen for more exciting developments in speech coding, no matter what algorithm you use….